Team from HTX creates first-of-its-kind dataset to tackle specular highlights in OCR and vision AI
Jovin Leong from HTX’s S&S CoE standing beside a display explaining the team’s method at NeurIPS 2024 in Vancouver, Canada. (Photo: HTX)
A team from HTX's
Sense-making & Surveillance (S&S) Centre of Expertise (CoE) has come up with a groundbreaking method of generating high-quality, real-world specular highlight datasets that would boost the training of vision AI.
The method was developed by a team consisting of lead engineers Jovin Leong, Ming Di Koa, Benjamin Cham, and Shaun Heng. Jovin even got to show off the fruits of their labour at the 38th Conference on Neural Information Processing Systems (NeurIPS 2024), which was held in Vancouver, Canada from 10-15 December.
NeurIPS is the premier conference for AI research publications in the world.
The inspiration for the idea behind this novel method came from S&S CoE’s collaboration with HTX’s
Chemical, Biological, Radiological, Nuclear, and Explosives (CBRNE) CoE, during which they learned of a problem faced during the scanning of parcel labels - plastic protective covers on parcels often result in bright spots or glare on images, known as specular highlights, leading to inaccuracies in optical character recognition (OCR) scanning.
Specular highlights are not only a problem for OCR – they are also an issue for computer vision systems that detect and recognise objects, such as vision systems on self-driving cars or robots.
To tackle this issue, the team knew it had to leverage datasets of images with specular highlights to train vision AI to remove or deal with these pesky bright spots. However, they found that such datasets are almost non-existent.
As such, the quartet decided to create their own datasets to address the challenge. To do so, they came up with a special setup that included:
- An enclosed box with LED lights and special filters that polarise the light
- A special camera sensor that can capture images at different polarisation angles
- Placing documents in transparent file folders with reflective surfaces
- Capturing images of the documents from four different images at once, each with a different polarisation angle
- Combining these images in ways that create both a normal image with specular highlights and a "clean" version without them
Thanks to this setup, the team created the SHDocs dataset, which contains over 3,000 document scenes and more than 19,000 images, including the pairs of original and “clean” images.
SHDocs is the first publicly available dataset in the world containing high-quality, real-world specular highlights on document images. The images in this dataset are high-quality and closely match real-world scenarios, and the pairing of each image with specular highlights with a perfectly aligned “clean” version is valuable for training AI models.
The team also developed a novel way to test how well different methods remove specular highlights from images, especially for document images with text. They first tried using standard image quality measures but found these didn't always match up with how humans would judge the image quality.
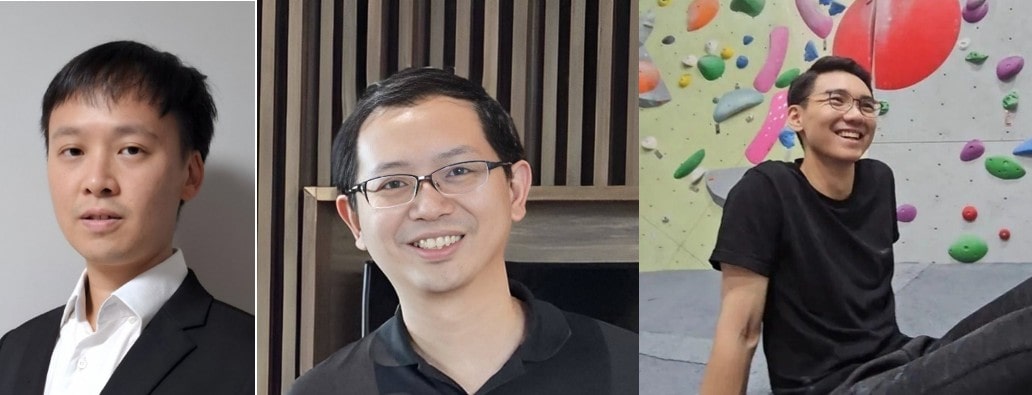
(left to right) S&S CoE engineers Benjamin Cham, Ming Di Koa and Shaun Heng. (Photo: HTX)
As such, they came up with a more practical test – using OCR software to see how well it could read the text in images after different specular highlight removal methods were applied.
With their new benchmark, the team discovered that some existing methods for removing specular highlights didn't work as well on document images as expected. Separately, the team also found that a comparatively simple AI models trained on the SHDocs dataset can outperform some of the leading specialised methods.
The team now hopes that the techniques developed can potentially be used by other researchers to create similar datasets for different types of images or applications. By making their dataset, code, and methods publicly available, the team hopes that the dataset created can catalyse the development new vision AI models that can be used to address the problem of specular highlights.